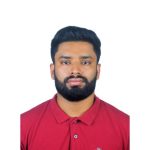
The project revolves around reproductive health and family planning. This is of vital importance, and has been indicated as one of the Sustainable Development Goals (SDGs) proposed by United Nation (UN) (PATH, 2019). Reproductive health and family planning is determined on a case-by-case basis, calculating client and community needs and program capabilities (Arlington, 2000). More concretely, the aim is to dramatically reduce maternal mortality, newborn and child mortality, and deaths from neglected diseases (PATH, 2019). The goal of this project is the betterment of family planning, in order to increase good health and well-being. Thus, this will provide a wide range of family planing chices and sexually transmitted infections (STIs) preventions (Arlington, 2000; USAID, 2022). The means of achieving this, lies in in global health supply chains, and more specifically, on the availability of contraceptives. This brings us to the topic of improved demand forecasting, which, if performed suboptimally, can lead to two societal consequences: first, overstock implies excess costs, and second, a stockout which can lead to the breaking of contraceptive habits and unwanted pregnancies. To achieve this improvement in demand forecasting, this study aims to create an innovative non-parametric machine learning approach to accurately forecast in the presence of low-quality data by feature engineering to select variables with high causal power rather than relying on historical consumption only.